Technology is reshaping how businesses operate on a day-to-day basis. Major companies are focusing on cost-effective automation processes, improving product quality and simplifying equipment maintenance. This technological shift has enabled manufacturers to ditch the reactive (run-to-failure) strategy in favor of predictive maintenance (PdM).
As it so happens, this transition also results in significant improvements to overall equipment effectiveness (OEE). Let’s see how.
The Impact of Predictive Maintenance on OEE
Predictive maintenance is a data-driven maintenance strategy that uses sensors to continuously monitor asset health and performance. It runs that data through a set of prebuilt predictive algorithms to estimate when a certain asset should experience failure. This enables maintenance teams to schedule preventive work just before failures occur, shortening and minimizing the number of both unscheduled and planned downtimes in the process.
PdM makes it possible for organizations to eliminate unnecessary breakdowns or production stops which are common with reactive maintenance strategies. It ensures that all the production assets will be available throughout the production cycles with minimum interference to production schedules. Increased availability of production assets significantly improves the Overall Equipment Effectiveness (OEE) for all production processes.
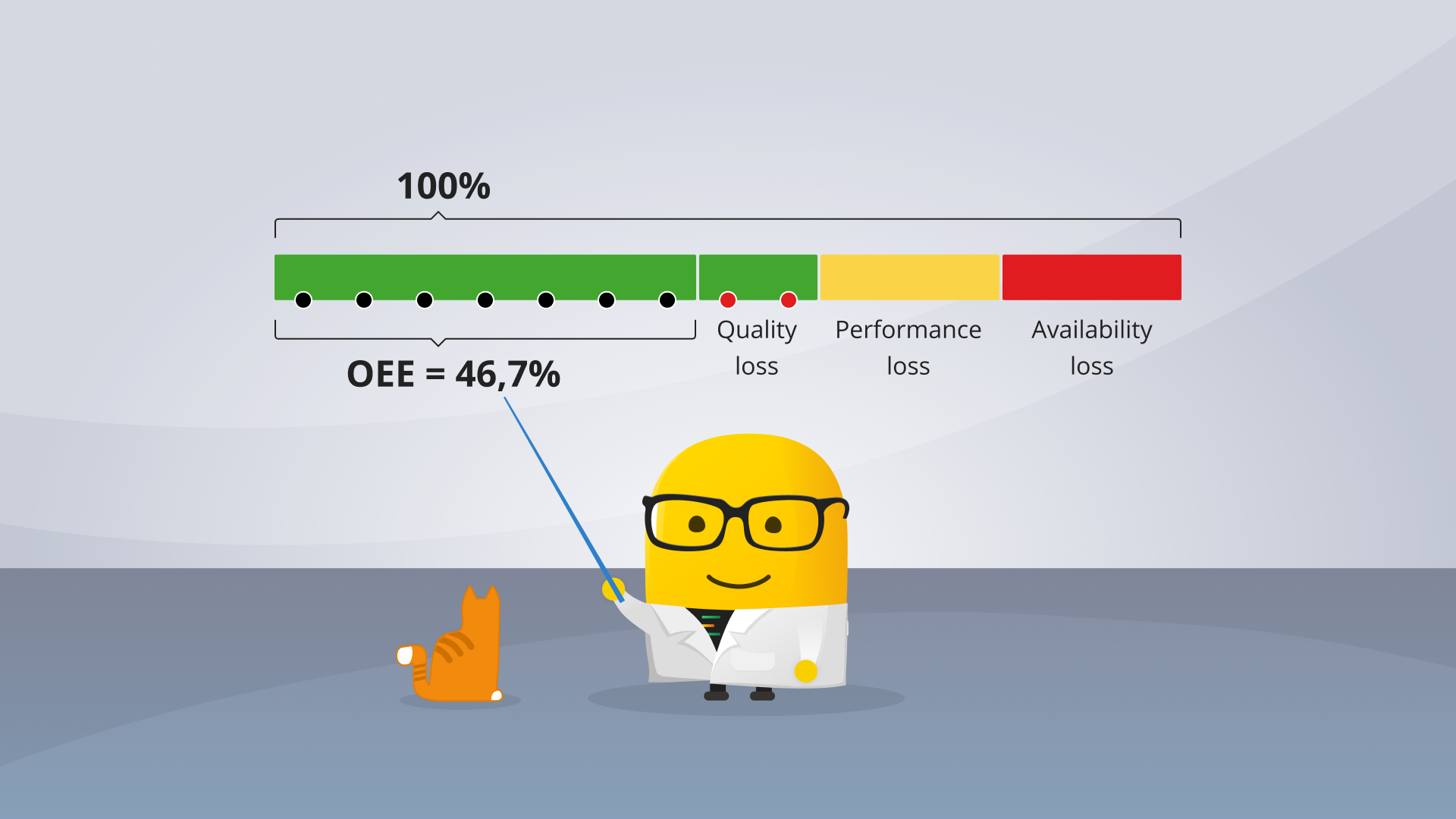
Implementation of a PdM program guarantees that the performances of all assets within a production facility are retained at their optimal levels which reduces the performance loss part of the OEE equation.
Instances of unprecedented equipment failure, reliance on worn-out, misaligned, defective or obsolete parts are also minimized. This leads to improvement in the quality of the end product. In other words, the number of defects is minimized as quality losses due to machine issues are significantly reduced.
Steps to Follow When Switching From Reactive to Predictive Maintenance
Aside from improving the OEE scores of a production facility, companies ought to reap many additional benefits by implementing PdM programs. Some of the notable impacts of PdM are improved operational safety, extended lifetime of critical assets, enhanced profitability, and better customer satisfaction.
Here is an outline of how to set up the transition from reactive to predictive maintenance.
1. Establish an implementation strategy
Before transitioning from a reactive program to a predictive program, a suitable implementation strategy has to be drawn up. The initial phase will involve a rigorous audit of all production assets to identify and prioritize equipment that should be included in the PdM program. Priority is given to assets with a history of frequent failure, critical assets (those that run round the clock), as well as expensive to replace and hard-to-access assets.
Upon identifying assets that need to be included in the pilot program, it is vital that previous maintenance data is scrutinized to create a preliminary predictive model and to conduct a detailed Failure Mode and Effect Analysis (FMEA) on assets.
A list of high-priority, high-risk equipment is generated after a detailed FMEA is conducted. The list is then leveraged to create and refine a pilot program by setting up milestones, devising evaluation methodologies, creating OEE improvement objectives, and defining data acquisition approaches.
2. Deploy additional infrastructure
Plenty of data has to be collected and fed into predictive algorithms so they can make accurate predictions. To achieve this, several condition monitoring sensors have to be fitted on the pilot equipment. These sensors collect data in real-time and transfer the information through dedicated IoT networks, to a centralized database for analysis. They can be used to measure a variety of different signals, from electrical currents and vibrations to noise and corrosion levels.
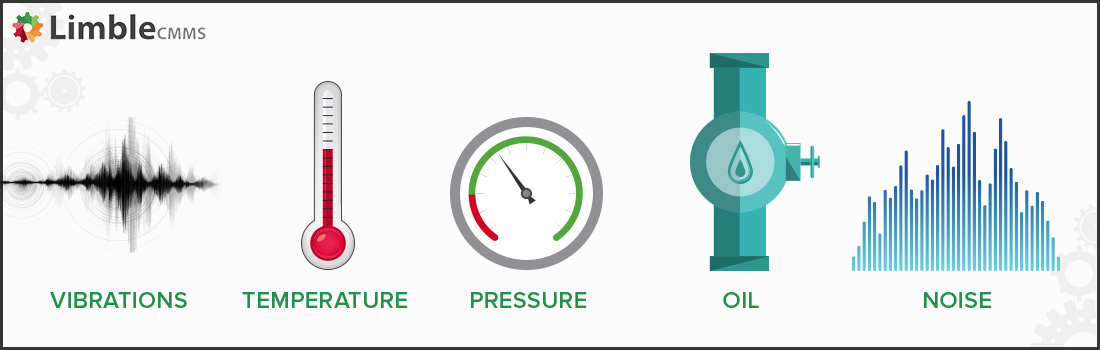
Based on this and previous maintenance data, a suitable prediction algorithm is developed. Advanced systems deploy AI and machine learning technologies at this stage. The algorithms counter-check supplied sensor data against preset conditions, generating alerts whenever deviations are identified.
To ease communications between machines and maintenance teams, a user-friendly dashboard is required. It is crucial that the data collected is protected against misuse or external attacks that may impede production cycles.
Start improving your OEE
Get all the tools you need to understand what's really happening on the shop floor in order to make improvements. Free for 30 days.
3. Train, test and gather feedback
Adopting a PdM program implies that a number of maintenance activities will undergo significant changes. For instance, certain manual readings are eliminated and new maintenance technologies, tools, and procedures are introduced to adjust to new procedures. The organization will also look to implement a CMMS that supports predictive maintenance or comparable software, if they don’t use one already.
While testing the pilot program, it is essential that all maintenance staff receive adequate training on newly implemented technology. During this window, they are informed of the changes in their roles and educated on how to navigate the new systems.
Maintenance teams will subject the pilot equipment to diverse production schedules and monitor their response to changes. As predictive algorithms are fed more data, their prediction will become increasingly accurate.
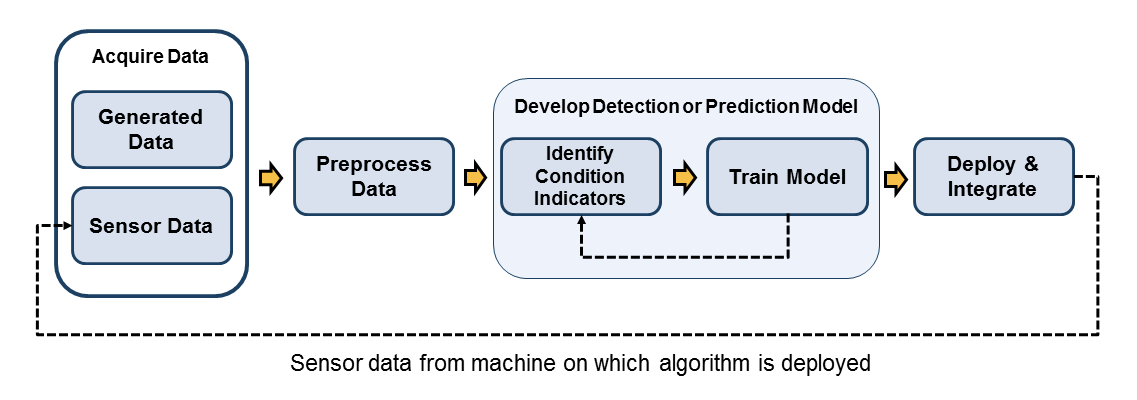
At this stage, the management receives feedback from the implementing teams to assess the ease of use of the PdM program and its contribution to improving OEE. Based on equipment performance data and personal feedback, the company can develop a robust response procedure to address alerts generated by the program.
4. Improve and scale-up the PdM program
It takes time for an organization to fully test and verify the contribution of a PdM program to their production schedule and output. The pilot program is subjected to diverse operational scenarios in an effort to extract a vast amount of actionable data. The pilot phase provides invaluable insights for the company to scale up or refine its PdM program.
Gaps identified during the test phase are rectified by refining the infrastructure or predictive algorithms. Once all issues have been resolved, the company can proceed to gradual, full scale implementation of the program. The scaling up of the program should be executed in stages and in a manner that avoids the overstraining of existing resources.
PdM programs require continuous lifetime upgrades for them to retain their relevance and competitiveness. The upgrades are vital for improving data security, increasing data acquisition and analysis speeds, and streamlining communications between human and machine interfaces.
Final Thoughts
PdM programs are cost-effective long-term solutions, especially when you consider OEE improvements they can bring. While PdM is an attractive strategy, the implementation should be carefully thought out to avoid common pitfalls.
The transition requires adequate planning and strategic execution to prevent production losses. High initial investment costs may be incurred with some processes requiring the input of experts (third-party service providers or in-house technicians). Despite the challenges, selecting the appropriate PdM model has the potential to convert manufacturing businesses into smarter, more profitable systems.
About the author: Bryan Christiansen is the founder and CEO of Limble CMMS. Limble is a modern, easy to use mobile CMMS software that takes the stress and chaos out of maintenance by helping managers organize, automate, and streamline their maintenance operations.